The Ethical Challenges of Artificial Intelligence and Machine Learning
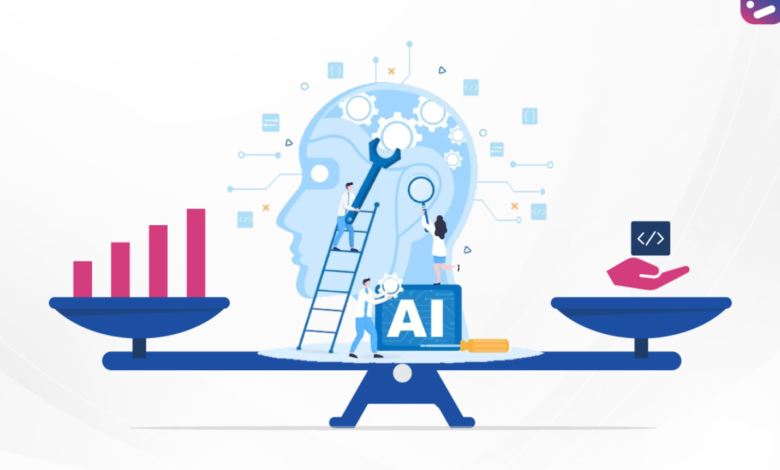
Artificial Intelligence (AI) and Machine Learning (ML) are transforming industries, improving efficiency, and unlocking unprecedented opportunities. However, these powerful technologies also pose significant ethical challenges. From concerns about privacy and fairness to accountability and societal impact, the ethical implications of AI and ML demand careful consideration and proactive regulation to ensure they serve humanity responsibly.
Understanding AI and ML Ethics
AI ethics is a set of principles and guidelines to govern the development and use of AI systems responsibly. ML, a subset of AI, involves algorithms that learn from data to make predictions or decisions, raising concerns about how the data is used and how these systems operate.
Key ethical principles include:
- Transparency: AI systems should be explainable and understandable.
- Fairness: Avoiding biases that discriminate against individuals or groups.
- Accountability: Ensuring responsibility for AI’s decisions and actions.
- Privacy: Protecting personal data and user rights.
- Security: Safeguarding AI systems from malicious use or tampering.
See also: Big Data and Analytics: Transforming Businesses in the Digital Age
Major Ethical Challenges in AI and ML
1. Bias and Discrimination
AI systems often inherit biases from the data they are trained on. This can lead to unfair outcomes, especially in areas like hiring, law enforcement, and lending.
Examples:
- Facial recognition systems showing higher error rates for people of certain races.
- Hiring algorithms favoring male candidates due to biased historical data.
Solutions:
- Diversifying training datasets to reflect a wider range of demographics.
- Regular audits to identify and mitigate bias in AI models.
2. Lack of Transparency (Black Box Systems)
Many AI models, especially deep learning systems, operate as “black boxes,” making decisions that are difficult to interpret. This lack of transparency creates challenges in understanding and trusting AI systems.
Impact:
- Difficulty in explaining decisions made by AI in critical applications like healthcare or finance.
- Limited accountability when errors occur.
Solutions:
- Developing explainable AI (XAI) to make decision-making processes more transparent.
- Requiring documentation and justification for AI-based decisions in sensitive fields.
3. Privacy Concerns
AI systems often rely on large datasets that include personal information. Improper use of this data can infringe on privacy and lead to misuse.
Examples:
- Social media platforms using user data for targeted ads without explicit consent.
- Surveillance systems collecting data on individuals without their knowledge.